RECOGNIZING FEATURES AND BEHAVIOR
In recent years, there has been a lot of discussion about Tesla’s choice to dedicate its resources in pursuing a vision-based approach to achieve full self-driving (FSD) instead of map based FSD making use of Light Detection and ranging (LiDar) technology. Tesla’s AI-based image analysis makes uses of complex Convolutional Neural Networks (CNN) algorithms. Neural networks can be trained to recognize and identify specific features of a captured environment. CNN can also be trained to decode how objects and human behave in that particular environment. A cyclist, for example, looking over his shoulder to make a turn, may already require the car to slow down. CNN algorithms can be trained to recognize such behavior when sufficient datasets with similar features are available. With each Tesla on the road gathering training data for the company’s AI team, the algorithms become more performant and new applications can be added every day. LiDar and other laser technology-based mapping solutions do not include all information needed for intelligent decision making. With laser signal-based mapping, it remains almost impossible to detect the intended maneuver of the same cyclist.
CNN: TRAINING ADDS TO FLEXIBILITY
Camco AI-based image analysis technology also relies on CNN algorithms as the obvious choice and primary information source: if visual recognition can do the job, then there is no need for (additional) expensive laser technology. Not only will this reduce costs, a vision-based solution will also increase product robustness and allow for more flexibility. However, it takes time and resources to develop the large data sets and train the right neural network before they can be integrated into products and solutions.
LARGE DATASET OF BoxCatcher IMAGES PERFECT BASIS FOR CNN TRAINING
Camco’s BoxCatcher is a sliding STS crane OCR system, capturing container data when loading or unloading ships. The intelligent camera system is linked to the crane PLC and travels along vertical mounted rails to the exact position where container cross the crane’s waterside legs. A camera-integrated laser triggers the camera when the container is best positioned for making pictures for data extraction. In the next generation BoxCatcher design, the laser has become redundant since its key triggering feature will have been integrated in the camera’s smarter neural network algorithm. Camco’s large database of BoxCatcher images indeed offers the ideal training set for building more complex and smarter neural networks. The additional CNN-based triggering feature overcomes the limitations of the laser-based solution where triggering can be affected by fog, dirt or salt residue on the (laser) cover and non-container shaped cargo.
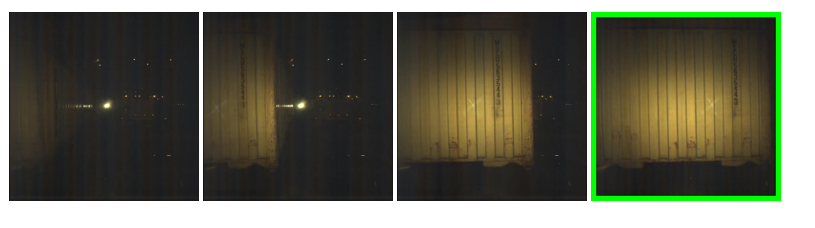
Series of images during night time crane operations, with moves up to 5m/s, to be processed by the AI engine in real-time for obtaining a centered and well captured container image.
AUTONOMOUS LPR WITH CNN
Camco’s streaming LPR OCR cameras no longer require a laser or radar system for signaling when a vehicle is approaching. Here again, Camco’s large LPR-based image dataset was used to train CNN algorithms into detecting approaching objects in the lane and identify cars, trucks or persons, all based on extracted features like the class, size and position of the object. This additional feature makes the LPR camera smarter and offers more flexibility in dealing with situations like queuing trucks, occlusion and bidirectional traffic, but also in ignoring certain vehicle types or changing the OCR trigger position on-the-fly. Last but not least, leaving the laser hardware out, makes the solution more robust and also less expensive.
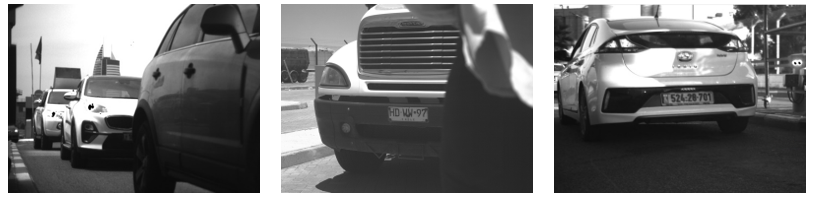
Streaming LPR can sometimes be challenging due to various external variables.